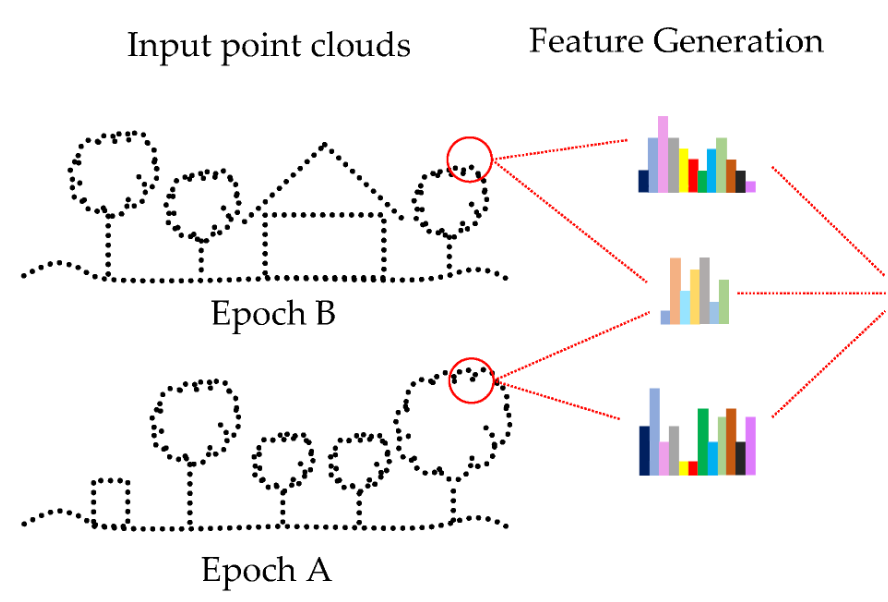
A review on 3D change detection using point clouds
A comprehensive review of the state of the art of 3D change detection approaches, mainly those using 3D point clouds.
Massive point clouds are being compiled quicker than ever before because to the quick growth of 3D data collecting, and they will soon serve as the primary topographic data for many applications
However, data must be updated and used to identify and define the changes that have taken place in a dynamic environment where everything is always changing.
Long-term changes may now be detected with better temporal resolution because to the constant availability of enormous volumes of satellite photos with quick return intervals. Similar to this, 3D change detection (3D CD) is becoming more and more popular as a result of the expansion of 3D data at various sizes (district, city, region, and country).
Massive point clouds are being compiled quicker than ever before because to the quick growth of 3D data collecting, and they will soon serve as the primary topographic data for many applications
However, data must be updated and used to identify and define the changes that have taken place in a dynamic environment where everything is always changing.
In remote sensing applications, CD has received much study, and several methods based on two-dimensional (2D) pictures have already been presented.
Similar to this, 3D change detection (3D CD) is becoming more and more popular as a result of the expansion of 3D data at various sizes (district, city, region, and country).
Depending on the researched phenomena, its frequency, amplitude, and velocity, change can range from rapid change (for instance, in the context of autonomous driving) to sluggish change (for example, remote sensing applications).
There are several ways to gauge and monitor each object's dynamics:
Land use and land cover change detection, urban monitoring, forest changes, crisis monitoring, 3D geographic information updating, landslide and erosion monitoring, construction progress monitoring, and resource surveying have all seen an increase in demand for 3D CD in 3D remote sensing applications.
Researchers provide a thorough analysis of 3D point cloud change detection in this publication. Without including the 3D scene flow or 3D object tracking methods, they analyzed the 3D CD techniques utilized in remote sensing applications.
They introduce learning-based and distance-based approaches, with a focus on deep learning-based approaches. The paper's four primary contributions are as follows:
- Issues with using point clouds in CD and an analysis of 3D CD techniques
- Detailed analysis of the most common point cloud datasets for 3D CD benchmarks
- Detailed explanation of assessment measures for determining the effectiveness of change detection
- A list of the issues still to be resolved and the areas that need further study to improve the development of CD employing 3D point clouds.
You can continue reading the research in the following article:
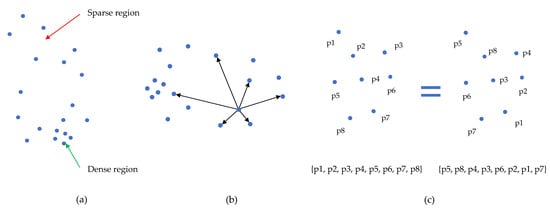
Abstract
Change detection is an important step for the characterization of object dynamics at the earth’s surface. In multi-temporal point clouds, the main challenge is to detect true changes at different granularities in a scene subject to significant noise and occlusion.
To better understand new research perspectives in this field, a deep review of recent advances in 3D change detection methods is needed. To this end, we present a comprehensive review of the state of the art of 3D change detection approaches, mainly those using 3D point clouds.
We review standard methods and recent advances in the use of machine and deep learning for change detection. In addition, the paper presents a summary of 3D point cloud benchmark datasets from different sensors (aerial, mobile, and static), together with associated information.
We also investigate representative evaluation metrics for this task. To finish, we present open questions and research perspectives. By reviewing the relevant papers in the field, we highlight the potential of bi- and multi-temporal point clouds for better monitoring analysis for various applications.
LiDAR INSIGHTER Newsletter
Join the newsletter to receive the latest updates in your inbox.